type
status
date
slug
summary
tags
category
icon
password
自然语言的出现是为了使人类的交流更加高效,自然语言处理(Natural Language Processing,简称NLP)致力于让计算机理解、解释和生成人类语言。
NLP结合了语言学、计算机科学和统计学的方法,以处理和分析大量的自然语言数据。它的主要目标是开发能够“理解”自然语言的计算机系统,使得它们能够执行诸如自动翻译、情感分析、信息检索和文本生成等任务。
语言模型
统计语言模型(Statistical Language Model)
统计语言模型是自然语言处理的基础模型,是从概率统计角度出发,解决自然语言上下文相关的特性的数学模型。统计语言模型的核心就是判断一个句子在文本中出现的概率。
假定S表示某个有意义的句子,由一连串特定顺序排列的词ω1, ω2组成,这里n是句子的长度。现在,我们想知道S在文本中出现的可能性,即S的概率P(S),则
P(S) = P(ω1,ω2,…,ωn) = P(ω1) ⋅ P(ω1|ω2) ⋅ P(ω3|ω1,ω2)…P(ωn|ω1,ω2…,ωn − 1)
即ωn依赖于前面n-1个ωi(i<n)
但这种方法太过复杂,因此需要简化该模型
Unigram language model
一元模型,即ωn 仅仅依赖于ωn − 1,但这样生成的文本只在相邻的两个字间有较大的关联性,长文本并无意义。
N-gram language model
假设ωn依赖于前面N-1个ωi(i<n)
当N=1时,即Unigram language model
当N=2时,Bigram Model
当N=3时,Trigram Model
…
Bag-of-Words
词袋模型(Bag-of-Words model,BOW)BoW(Bag of Words)词袋模型最初被用在文本分类中,将文档表示成特征矢量。它的基本思想是假定对于一个文本,忽略其词序和语法、句法,仅仅将其看做是一些词汇的集合,而文本中的每个词汇都是独立的。简单说就是讲每篇文档都看成一个袋子(因为里面装的都是词汇,所以称为词袋,Bag of words即因此而来),然后看这个袋子里装的都是些什么词汇,将其分类。



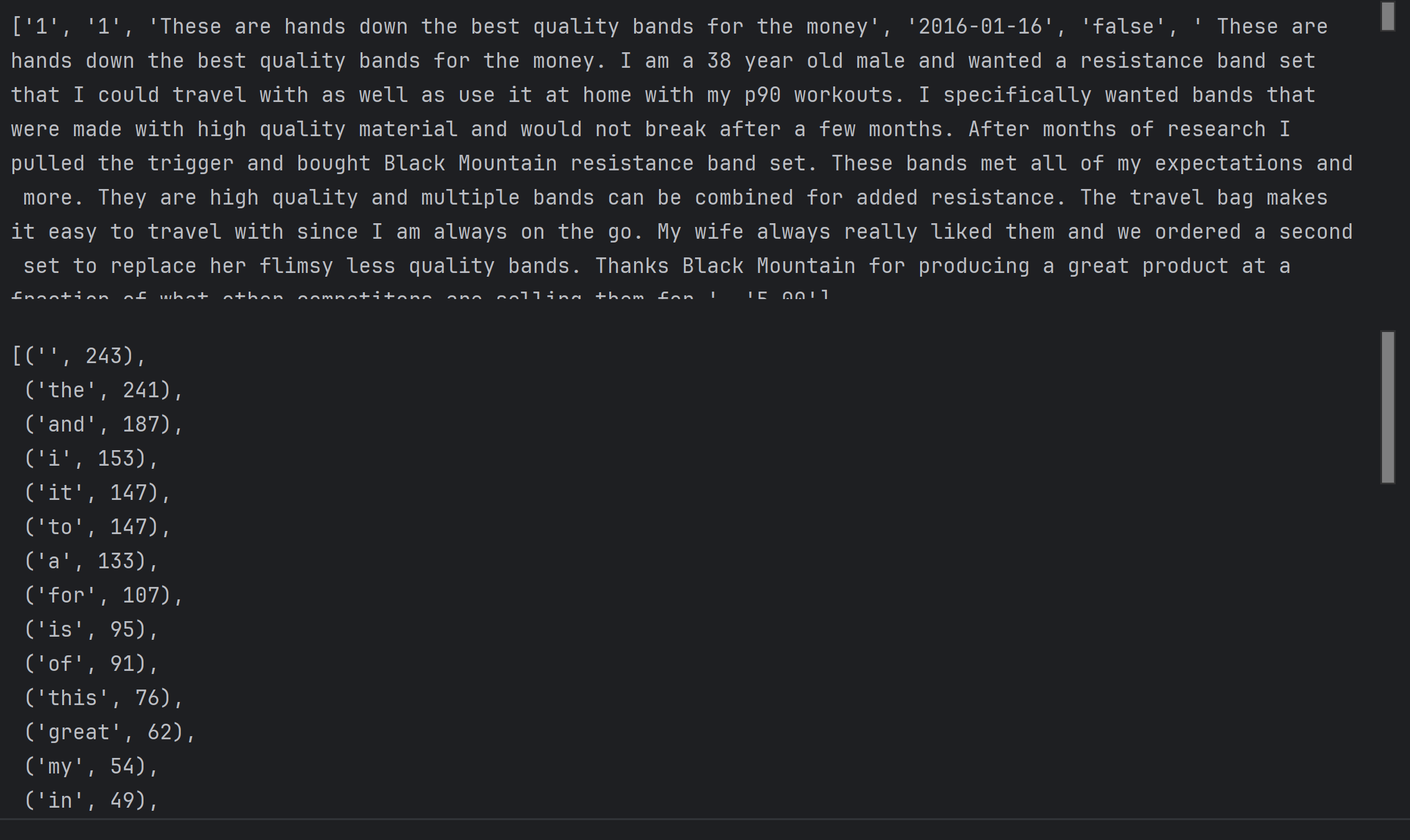
错误解决:在运行tokenize.word_tokenize()方法时出现了报错Resource punkt not found. Please use the NLTK Downloader to obtain the resource
经检查,nltk包已经成功地导入了,根据报错信息了解到是加载不到tokenizers/punkt/english.pickle文件在网上寻找到了解决方法,需要重新下载nltk_data,在nltk_data下的packages下找到tokenizers/punkt,解压缩后把tokenizers文件夹放到nltk_data-gh-pages文件夹下面,因为我使用了anaconda,最后将nltk_data放在了相对应的envs路径下,便解决了问题或者直接运行nltk.download('punk')
语句,但可能因为网络问题会出现下载失败的情况(也许修改使用国内镜像会有帮助)
向量空间模型(vector space model)
向量空间模型概念简单,把对文本内容的处理简化为向量空间中的向量运算,并且它以空间上的相似度表达语义的相似度,直观易懂。当文档被表示为文档空间的向量,就可以通过计算向量之间的相似性来度量文档间的相似性。
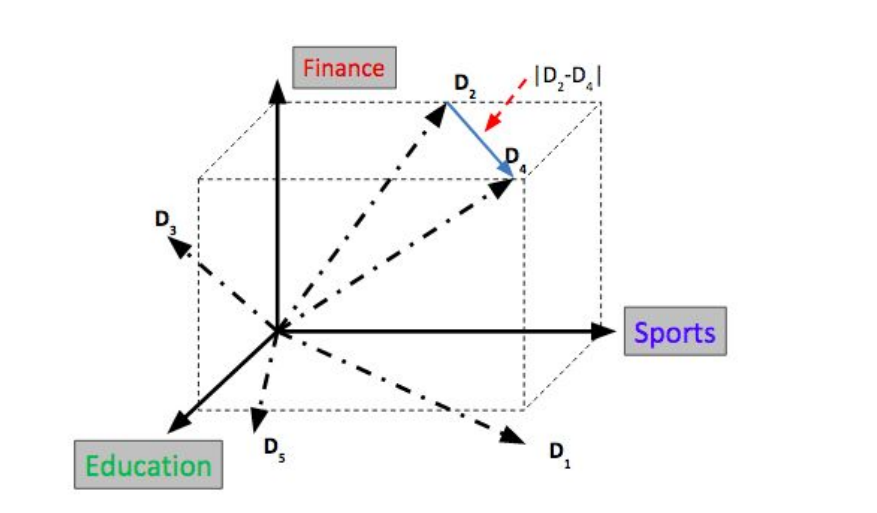
NLP Pipeline
文本分割(Segmentation/Tokenization):
将一段连续的文本分割成有意义的单位,如单词、短语、符号等。
示例:输入句子 “Text mining is to identify useful information.”,输出结果为 [“text”, “mining”, “is”, “to”, “identify”, “useful”, “information”]。
去除停用词(Stop Word Removal):
停用词是指那些频率很高但没有实质内容的词,如 “the”, “is”, “in” 等。去除这些词可以减少词汇表的大小,使后续的分析更加高效。
示例:输入 [“text”, “mining”, “is”, “to”, “identify”, “useful”, “information”],输出结果为 [“text”, “mining”, “identify”, “useful”, “information”]。
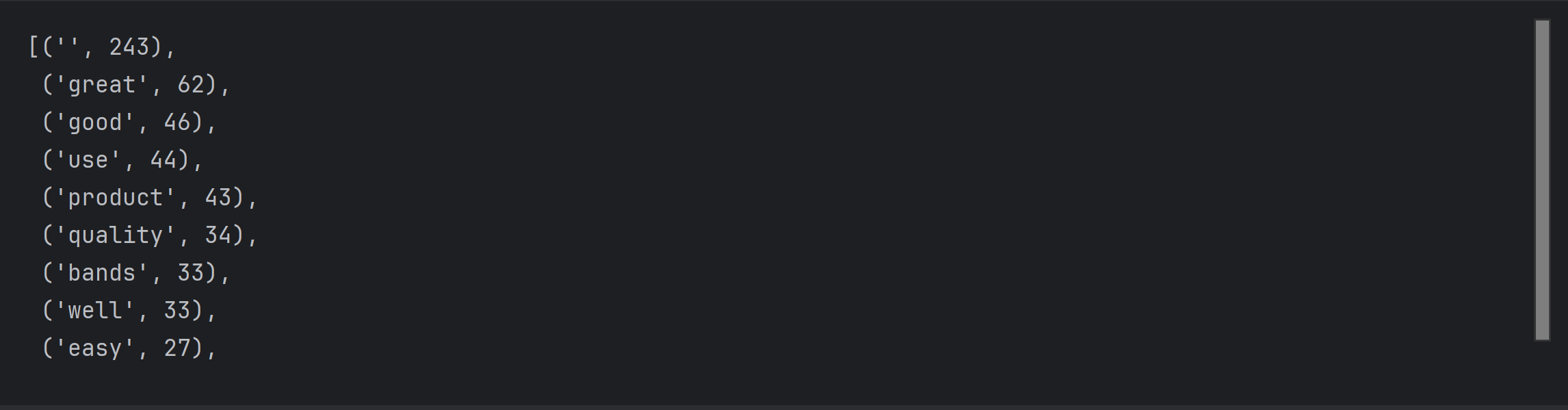
词干提取(Stemming):
将单词还原到其词干形式,即去掉单词的词缀。
示例:输入 [“text”, “mining”, “identify”, “useful”, “information”],输出结果为 [“text”, “mine”, “identify”, “use”, “inform”]。

词袋模型(Bag-of-Words):
将文本转化为词频向量,不考虑词的顺序,仅统计每个词出现的频率。
TF-IDF
TF-IDF(term frequency–inverse document frequency,词频-逆向文件频率)是一种用于信息检索(information retrieval)与文本挖掘(text mining)的常用加权技术。
TF-IDF是一种统计方法,用以评估一字词对于一个文件集或一个语料库中的其中一份文件的重要程度。字词的重要性随着它在文件中出现的次数成正比增加,但同时会随着它在语料库中出现的频率成反比下降。
TF-IDF的主要思想是:如果某个单词在一篇文章中出现的频率TF高,并且在其他文章中很少出现,则认为此词或者短语具有很好的类别区分能力,适合用来分类。
词频(TF)表示词条(关键字)在文本中出现的频率。这个数字通常会被归一化(一般是词频除以文章总词数), 以防止它偏向长的文件。
即
逆向文件频率 (IDF) :某一特定词语的IDF,可以由总文件数目除以包含该词语的文件的数目,再将得到的商取对数得到。如果包含词条t的文档越少, IDF越大,则说明词条具有很好的类别区分能力。
即, 分母之所以要加1,是为了避免分母为0
TF-IDF实际上是:TF * IDF 即
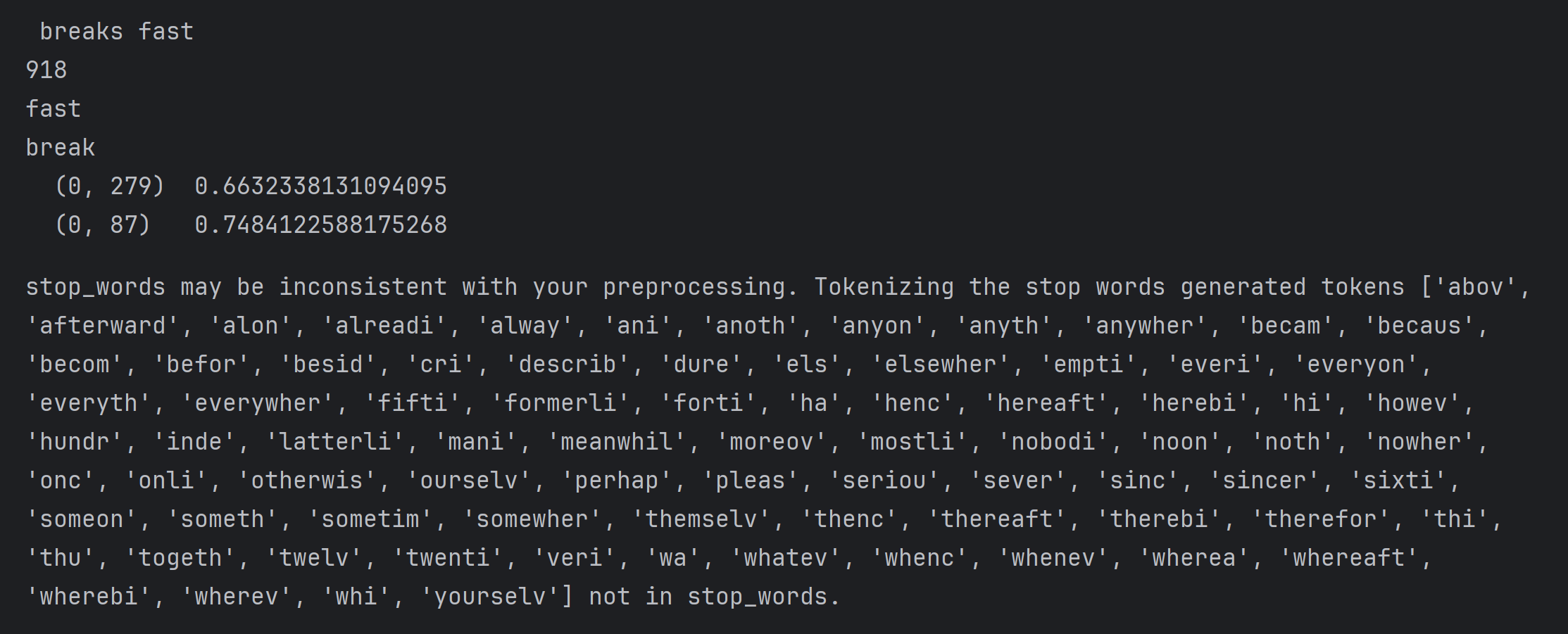

Text Classification 文本分类
Binary classification:只有两个类别
K-category classification: 超过两个类别(k个类别)
重点讲解Machine Learning的求解方法
Logistic Regression 逻辑回归
逻辑回归的原理是用逻辑函数把线性回归的结果(-∞,∞)映射到(0,1)
Support Vector Machine 支持向量机
是一种二分类模型,它的目的是寻找一个超平面来对样本进行分割,分割的原则是间隔最大化,最终转化为一个凸二次规划问题来求解。
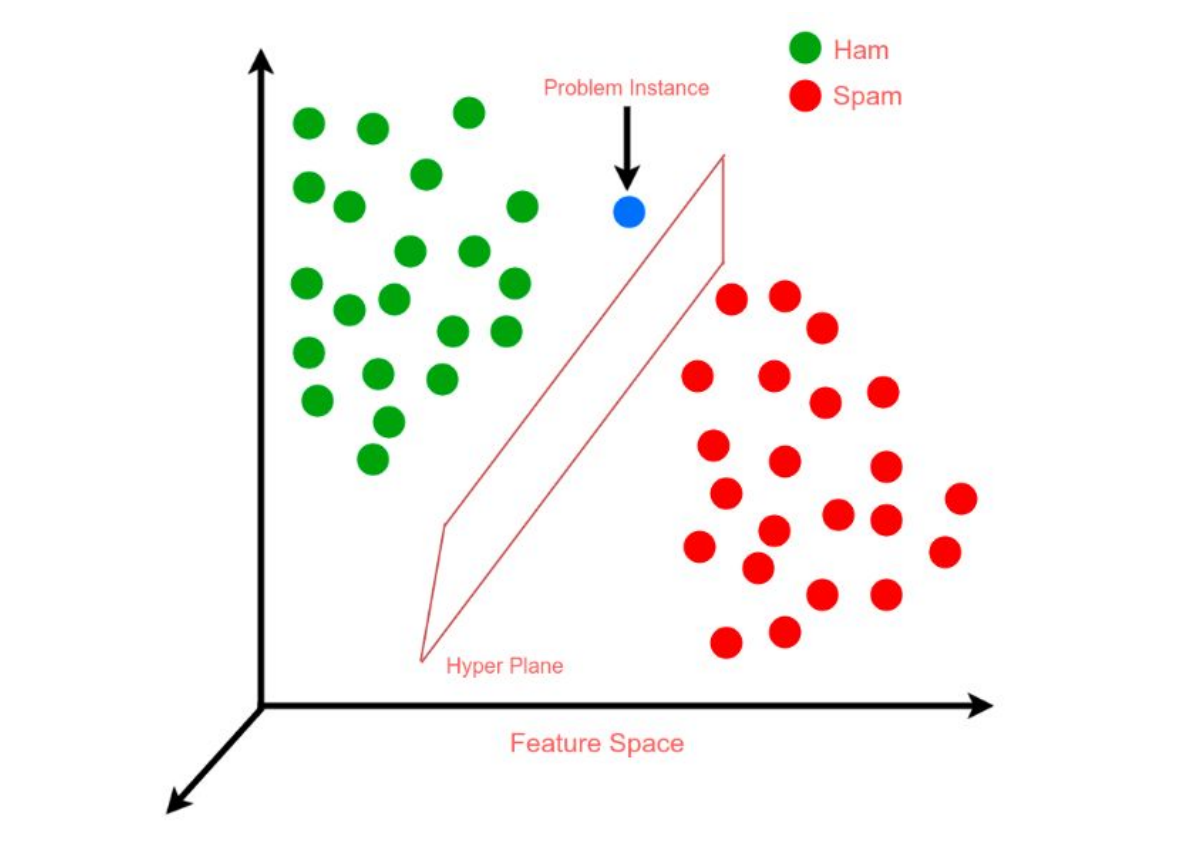


- 作者:Rainnn
- 链接:https://tangly1024.com/article/3523d0dc-5474-46f4-b6ce-c82187489018
- 声明:本文采用 CC BY-NC-SA 4.0 许可协议,转载请注明出处。